
Introduction:
Two of the most important mathematical representations are vectors and matrices from linear algebra. Vectors are often representations of positions or directions in two or three dimensions of space, but can also represent other quantities like sensor measurements. Matrices are representations of how representations change, either through an action, or even through a change in how those numbers are interpreted. We will be using them liberally throughout the book, and they appear in almost every subject of robotics. Hence, they must be mastered to get anywhere beyond a superficial understanding of the material.

1. Vectors and coordinates :
Vectors extend concepts that are familiar to us from working with real numbers âďż˝ to other spaces of interest. They also succinctly represent collections of real numbers that have a common meaning like position or direction, or readings from a signal taken at a given time. They make mathematical expressions more compact, which helps us wrap our heads around more difficult concepts.
Most often, đ-dimensional Euclidean spaces âđ is used, in which a vector is simply a tuple of đ real numbers. The "list of numbers" interpretation is the most common way that vectors are conceived of by engineers and computer scientists, and that is certainly how they are stored and operated upon. Let us call this the "layman's definition" of vectors. However, it is often important to realize that these numbers are just an interpretation of a more abstract essential concept -- the underlying physical meaning -- and the numbers will change depending on their manner of interpretation, such as a chosen frame of reference. This section will present common operations in 2D and 3D, and follow it with a discussion about the importance of separating meaning from representation.
1.1 2D coordinate frames:
In the "layman's definition", an đ-dimensional vector đą is a tuple of real numbers đą=(đĽ1,âŚ,đĽđ)ââđ. For now, we will work in â2. We will use boldface notation only temporarily to help distinguish between vectors and real numbers. In the future, the boldface will typically be dropped.
A 2D position đ is represented by a 2-element vector đŠ=(đđĽ,đđŚ) that gives its coordinates relative to axis directions đ and đ, offset from a position đ where the axes cross, called the origin We will also represent vectors in column vector form

for use in matrix-vector products. Both parenthetical and column vector notations are equivalent and interchangeable.
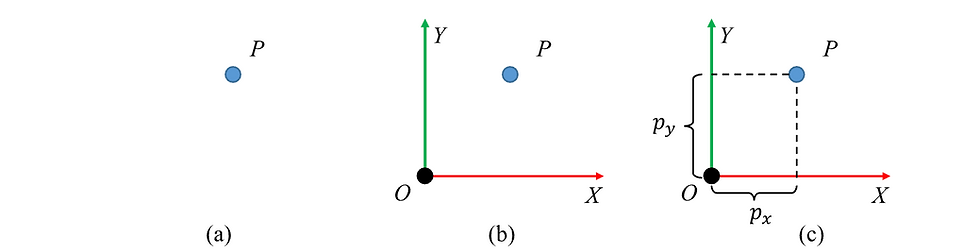
Figure 1. A point đ in the plane (a) has no numerical representation until we define a reference coordinate frame (b), which has origin point đ and orthogonal coordinate axes đ and đ. Its coordinates đŠ=(đđĽ,đđŚ) are respectively the extents of đ along đ and đ from the origin (c).
The items đ, đ, and đ define the coordinate frame in which the coordinates are interpreted. Here đ is an arbitrary position in space, and đ and đ are orthogonal directions with đ rotated 90â90â counter-clockwise from đ. Note that in isolation, a vector of coordinates does not define a position. A physical position is only defined by coordinates in reference to a certain coordinate frame. The frame will often be left implicit, or spoken of as the reference frame of the coordinates
1.2 3D coordinate frames:
The situation in 3D space is similar, except that we represent a 3D position đ with a 3-element vector đŠ=(đđĽ,đđŚ,đđ§)that gives its coordinates relative to axes đ, đ, and đ and offset from an origin đ in 3D space where the axes cross. The parenthetical notation is equivalent to the column vector form:
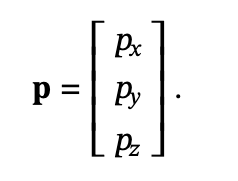
In 3D the coordinate frame consists of the origin đ and the mutually orthogonal axes đ, đ, and đ. In this book we will use right-handed coordinate convention in which the axes can be envisioned in the layout of the first three fingers of the right hand, suitably arranged at 90â90â right angles. đ axis corresponds to the thumb, đ axis corresponds to the index finger, and đ axis corresponds to the middle finger.
1.3 Directional quantities :
Vectors are also used to represent directional quantities, such as a displacement, direction, or derivative. A displacement is a difference between points, e.g., đŞâđŠ gives the amount that would need to be moved in both the đ and đ direction to move from đ to đ, where đŞ gives the coordinates of đ relative to the same reference frame. It has both a direction and a magnitude. In contrast, a direction does not have magnitude, and is a unit vector. The direction from đ to đ is given by
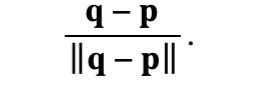
In 2D, a direction can also be given as an angle đâ[0,2đ)rad, with the convention that the angle measures the counter-clockwise direction from the đ axis. The corresponding unit vector is (cos(đ),sin(đ)).

Figure 2. Directional quantities arise from displacements (a), directions (b), and derivatives of paths (c).
A derivative is an infinitesimal displacement. If the position đ(đĄ) is a function of đĄ, then its derivative đŠâ˛(đĄ)is a vector (đđĽ(đĄ),đđŚ(đĄ)).
The major difference between directional and position quantities is that coordinates of directional quantities do not vary with respect to the choice of origin. However, coordinates of both positions and directions are affected by the choice of coordinate axes.
1.4 Geometric operations :
The coordinates of a point đŠ after translation by a displacement đ can be computed by vector addition đŠ+đ. Interpolation and extrapolation between points đŠ, đŞ is specified by the equation
đą(đ˘)=(1âđ˘)đŠ+đ˘đŞ(4)
for đ˘ââ. This equation starts at đą(0)=đŠ at đ˘=0, and ends at đą(1)=đŞ at đ˘=1. Extrapolation can be obtained with đ˘<0 or đ˘>1, as shown in Figure 3.
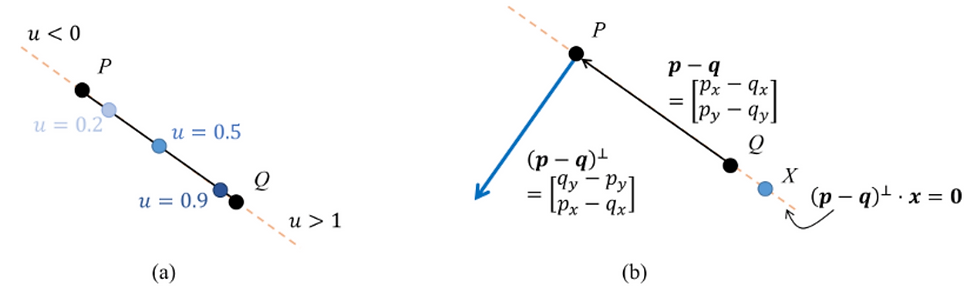
Figure 3. The line passing through points đ and đ can be modeled as a parametric interpolation (a) or as a plane equation (b).
The line through đŠ and đŞ can be obtained by sweeping the above interpolation / extrapolation formula across the entire range of đ˘ââ. The line segment between đŠ and đŞ is obtained by sweeping đ˘ across the range [0,1][0,1].
An other useful definition of a line in 2D uses a point on the line and an orthogonal direction. We define đŠâĽ=(âđđŚ,đđĽ) as an orthogonal direction to đŠ=(đđĽ,đđŚ), which has the same magnitude but is rotated 90 clockwise. The line through the origin passing through đŠ can be expressed in the form of all solutions đą to the equation đąâ đŠâĽ=0. Similarly, the line through points đ and đ can be expressed as the equation
đąâ (đŠâđŞ)âĽ=đŠâ (đŠâđŞ)âĽ
Another expression of lines is the following:
đąâ đ§=đ
Where đ§ is orthogonal to the direction of the line and đ=đŠâ đ§ for any point đŠ on the line. (Fig. 3.b.)
This definition is known as the plane equation, which generalizes lines in 2D to planes in 3D and hyperplanes in higher dimensions. Each of these is object of đâ1 dimensions in an đ-dimensional space, which we call a generalized plane. A unique representation for a generalized plane is đąâ đŽ=đ where đŽ is a unit vector orthogonal to the plane known as the normal direction and đ is a nonnegative offset that determines the distance away from the origin.
2. Transformations:
Transformations are functions that map đ-D vectors to other đ-D vectors: đ:âđââđ. They can represent geometric operations, which are caused by movement or action, as well as changes of coordinates, which are caused by changes of interpretation. Many common spatial transformations, including translations, rotations, and scaling are represented by matrix / vector operations. Changes of coordinate frames are also matrix / vector operations. As a result, transformation matrices are stored and operated on ubiquitously in robotics.
2.1 Linear transformations:
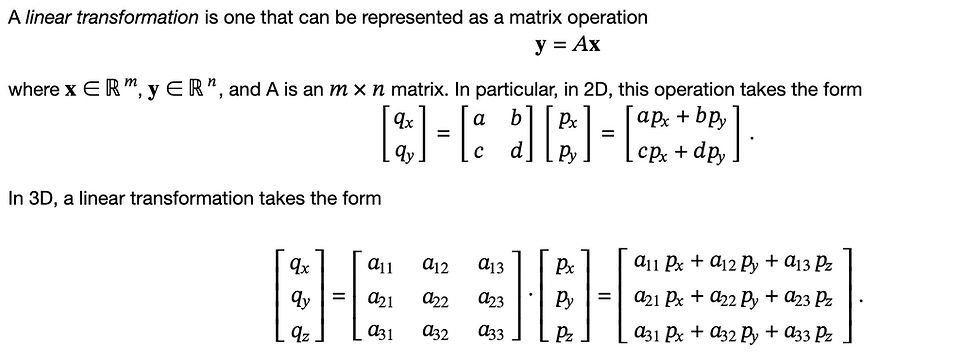
2.2 Rotations in 2D
Rotations about the origin by angle đ can be defined as linear transformations. Consider two reference frames with a common origin đ, the pre-rotation axes đ and đ, and the post-rotation axes đⲠand đâ˛. Depicting đⲠon top of đ and đ as a line emanating from the origin, and using a little trigonometry, we shall see that đâ˛has coordinates đąâ˛=(cosđ,sinđ). It is a bit more involved, but not much, to determine that đⲠhas coordinates đ˛â˛=(âsinđ,cosđ).
Now consider that along with the coordinate frames, a point đ was rotated to đâ˛. We will derive how to determine its new coordinates relative to the original reference frame. Notice that đⲠstill has coordinates (đđĽ,đđŚ)relative to the post-rotation frame đâ˛, đâ˛, since distances do not shrink or grow when objects are rotated. Specifically, đⲠis obtained by walking đđĽ units from the origin in the direction of đâ˛, and then đđŚ units in the direction of đⲠ(Fig. 4). Hence, to determine its coordinates in the original reference frame, we can use the fact that the coordinates of đⲠand đⲠare known.

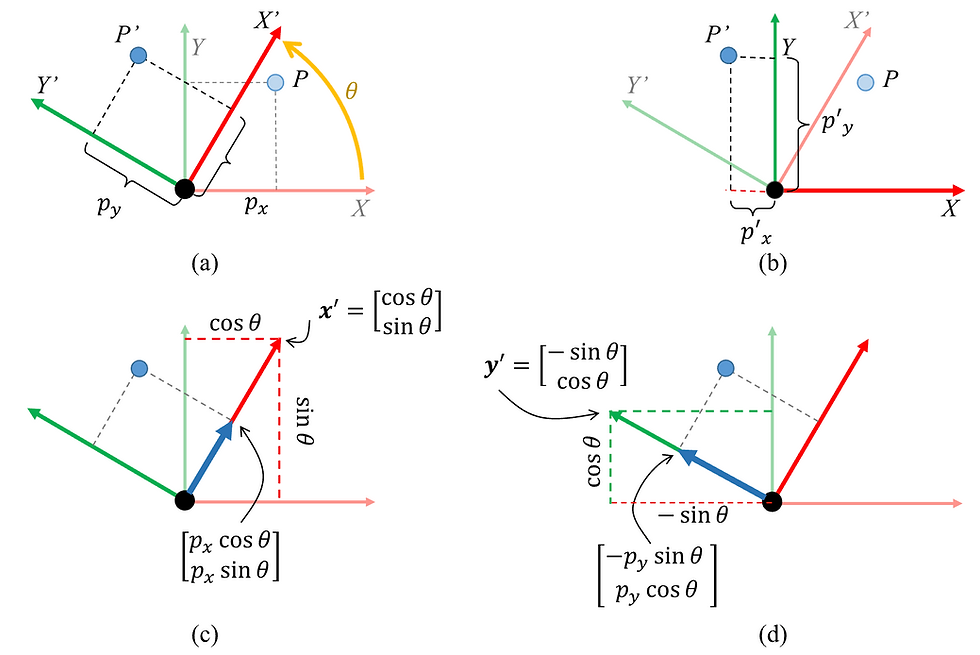
Figure 4. Rotating at an angle đ about the origin to achieve a new point đâ˛(a). To calculate the coordinates of đⲠ(b), we first obtain the coordinates of transformed axes đⲠand đâ˛(c,d).
A more compact and convenient way of writing this is with a matrix equation
đŠâ˛=đ (đ)đŠ
with the rotation matrix given by:
đ (đ)=[cosđsinđâsinđcosđ].
There are several useful properties of such matrices:
The matrix composition đ (đ1)đ (đ2)=đ (đ1+đ2) gives the rotation matrix for the sum of the angles.
The determinant det(đ (đ))=cos2đ+sin2đ for all đ.
Due to the identities cos(âđ)=cos(đ) and sin(âđ)=âsin(đ), the operation of rotating about âđ is equivalent to a matrix transpose: đ (âđ)=[cosđâsinđsinđcosđ]=đ (đ)đ.
Moreover, the transpose is equivalent to the matrix inverse: đ (âđ)=đ (đ)đ=đ (đ)â1. In other words, rotation matrices are orthogonal.
Vector norms are invariant under rotation: âđ (đ)đąâ=âđąâ.
The rotation matrix is only dependent on the argument's value modulo 2đ.
The space of rotations is known as the special orthogonal group đđ(2). The reason why it is called the special orthogonal group is that it is the set of all orthogonal 2Ă22Ă2 matrices with positive determinant, while there do exist other orthogonal matrices with determinant -1.
Property 4 implies that it is more proper to consider rotation matrices as only representing instantaneous orientation rather than accumulated amounts of revolution. For example, if a motor has spun 720â, the matrix representation is indistinguishable from the 0 rotation. In certain applications that demand reasoning about accumulated revolution, the representation đâ is more appropriate than a matrix. More about this topic will be discussed when we cover topological spaces.
2.3 Rotations in 3D
In 3D, rotations can also be defined as linear transformations, although parameterizing them is not as simple as in 2D. 3D rotation representations will discussed in further detail, but for now let us describe some of their properties.
A rotation in 3D can be represented by a matrix equation
đŠâ˛=đ đŠ
with đ a 3Ă33Ă3 rotation matrix.
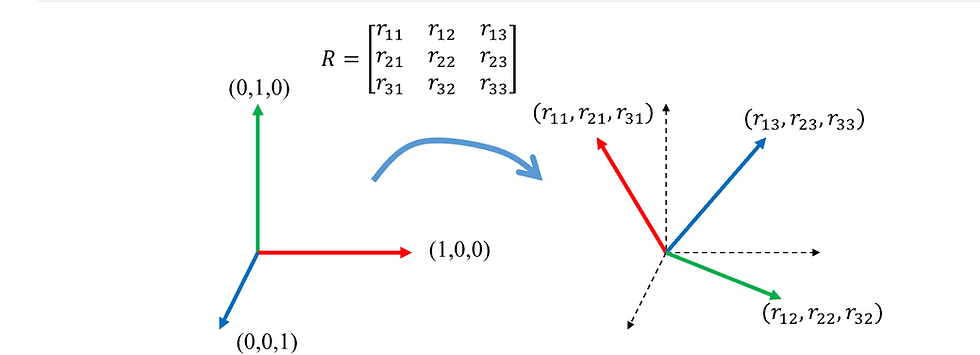
Figure 5. A 3D rotation is encoded by a 3Ă33Ă3 matrix whose columns give the coordinates of the rotated axes relative to the original axes.
This interpretation is useful when determining the coordinates of a rotated point in the original reference frame: if the point is given by coordinates đŠ=(đđĽ,đđŚ,đđ§) such that đâđ=đđĽđ+đđŚđ+đđ§đ, then the new coordinates of đ relative to the old reference frame are given by đ đŠ.
2.4 Scaling
Axis-aligned scaling in 2D about the origin can be represented as a linear transform with matrix.
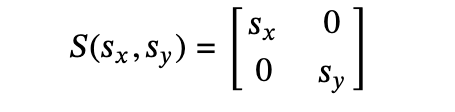
where đ đĽ is the scaling about the đ direction and đ đŚ is the scaling about the đ direction. If đ đĽ=đ đŚ this is known as a uniform scaling.
This definition can be generalized to đ-D space using an đ-D scaling vector đŹ which determines the scaling in each direction by mapping to the diagonal of an đĂđ matrix:
đ(đŹ)=đđđđ(đŹ).
2.5 Compositions of linear transformations
When performing two linear transformations one after another, the results are determined via matrix multiplication. Suppose that đ1(đą) and đ2(đą) are both linear transformations with matrices đ´ and đľ, respectively. When performing the operation of đ2 first to obtain đ˛=đ2(đą), then performing đ1 to obtain đł=đ1(đ˛), the ultimate result is:
đł=đ1(đ2(đą))
where it should be noted that đ1appears first in the equation even though it is performed after đ2. Expanding this into matrix products,
đł=đ´đľđą
holding for all values of đą. As a result, the function composition đ1âđ2 is also a linear transformation with matrix đ´đľ.
Using composition we can derive other useful transformations, like scaling not aligned to an axis. Suppose we wish to scale some coordinates by value đ in a direction đŻ, where đŻ is a unit vector. We can think of this as first rotating by an angle đ so that the đ axis is aligned with đŻ, then performing an axis-aligned scaling, then rotating back to the original coordinate frame:
đ´=đ (âđ)đ(đ ,1)đ (đ).
It so happens that

Note that due to the non-symmetricity of matrix multiplication, order of transformation matters: a rotation followed by a scaling is not necessarily the same as a scaling followed by a rotation. However, with a little inspection, we can derive the following symmetric compositions:
As a consequence of đ (đ1)đ (đ2)=đ (đ1+đ2), rotation by angle đ1 followed by angle đ2 is symmetric: đ (đ1)đ (đ2)=đ (đ2)đ (đ1). (Note that symmetry does not generally hold in 3D!)
A rotation and a uniform scaling.
Two axis-aligned scalings.
2.6 Rigid transformations
Rigid transformations in two dimensions have two properties:
The distance between two points do not change after being transformed.
In 2D, the orientation and area of any triangle does not change, and in 3D, the orientation and volume of any tetrahedron does not change.
The form of all rigid transforms is a rotation đ ďż˝ followed by an arbitrary translation đ:
đ(đą)=đ đą+đ(24)(24)
Which can be thought of applying a rotation about the origin first, and then a translation second. Proving that all rigid transforms have this form will be left as an exercise.
It is also possible to interpret rigid transforms as rotation about an arbitrary point. Letting the center of rotation be denoted đ, a rotation about đ can be constructed by translating a point so that đ is the origin, then rotating about the origin by some matrix đ , and then translating back to the original origin. This form is:
đ(đą)=đ (đąâđ)+đ.
The parenthetical term is the translation to đ as the origin, the multiplication by đ ďż˝ is the rotation about the new origin, and the addition of đ is the translation back to the original origin. These two representations are related by đ=đâđ đ, and đ=(đźâđ )â1đ.
The set of rigid transformations is called the special Euclidean group đđ¸(2) in 2D, and đđ¸(3) in 3D. Repeated application of rigid transformations also produce a rigid transformation. Given two rigid transforms
đ1(đą)=đ 1đą+đ1
and
đ2(đą)=đ 2đą+đ2
then the composite transform đ1âđ2 is the operation of performing đ2 first, then đ1. If we let đ˛=đ2(đą), and đł=đ1(đ˛), then we obtain:
đł=đ1(đ2(đą))=đ 1(đ 2đą+đ2)+đ1.
By the distributive property of matrix multiplication, we have
đł=đ 1đ 2đą+đ 1đ2+đ1.
This is simply a rigid transform with rotation matrix đ 1đ 2 and translation by (đ 1đ2+đ1).
2.7 Inverse transformations
Not all transformations have inverses, but rotations, translations, rigid transformations, and many linear transformations do. As described before, the inverse of a rotation matrix is simply its transpose. Translations are inverted by translating in the negative direction. Linear transforms đ´đą are invertible only if the matrix is invertible, with the inverse transformation đ´â1đą.
Rigid transformations are also invertible, and their inverse is also a rigid transformation:
đâ1(đ ,đ)=đ(đ đ,âđ đđ)
where đ(đ ,đ)(đą)=đ đą+đ. Proof of this equation will be left as an exercise.
2.8 Rigid movement
Rigid transformations are used to represent movement of rigid bodies in space. If, in 2D the origin of a body moves by translation đ in its original reference frame and rotates by angle đ =đ (đ), then the transformation that converts positional coordinates from the new coordinate frame to the original coordinate frame is given by đđ(đą)=đ đą+đ. In other words, if đą gives the coordinates of a position đ that is attached to the body, then after moving, đ will have coordinates đđ(đą) relative to the original body's frame. However, the transformation of directional coordinates will simply be a rotation and ignore translation: đđ(đŻ)=đ đŻ. In other words, if đŻ gives the coordinates of a directional quantity đ that is attached to the body (such as the direction of a line attached to the body), then đ will have coordinates đđ(đŻ) relative to the original coordinate frame (as in other directional quantities, these are interpreted ignoring the origin).
2.9 Representation of coordinate frames and coordinate transforms
Coordinate frames, as well as conversions between them, are interpreted as rigid transformations.
Any 2D coordinate frame đš with origin đ and axes đ and đ may be represented by the coordinates of đ, đ, and đ in some privileged world frame. If đ has coordinates đ, and đ and đ have (directional) coordinates đą=(đĽ1,đĽ2) and đ˛=(đŚ1,đŚ2) relative to đ, then the world coordinates of any point đ such that đŠ is its coordinates in the frame đš can be calculated by the rigid transform

because đ and đ are both orthogonal and đ is 90 ccw from đ.) The information stored for a 3D coordinate frame is similarly a rotation matrix đ and origin coordinates đ. This operation is known as the coordinate transform đ´âđ with đ´ the source frame and đ the target frame . We can also perform the reverse coordinate transform from đâđ´ by applying the inverse transform. Changes of coordinate frames can also be represented in terms of rigid transforms. Suppose đ´ and đľ are two coordinate frames, where đ´ is represented with respect to the world frame by a rotation matrix đ đ´ and translation đđ´, and đľ is represented by đ đľ and đđľ. Then given the coordinates đŠđ´ of some point đ relative to đ´, we can determine đ's coordinates relative to đŠđľ in two steps. First, we calculate its world coordinates:
đŠđ=đđ´(đŠđ´)=đ đ´đŠđ´+đđ´
And then we perform the inverse of đľ coordinates to world coordinate to obtain its coordinates with respect to đľ:
đŠđľ=đâ1đľ(đŠđ)=đ đđľ(đŠđâđđľ).
This transform can be calculated for all points by the composition of the transform from đ´âđ and then đâđľ: đŠđľ=đâ1đľ(đđ´(đŠđ´))=đ đđľđ đ´đŠđ´+đ đđľ(đđ´âđđľ).
2.10 Homogeneous coordinate representations
Homogeneous coordinates gives a convenient representation of rigid transforms as linear transforms on an expanded space. Moreover, it compactly represents the distinction between positional and directional quantities. The idea is to augment every point with an additional homogeneous coordinate, which is 1 if it is positional and 0 if it is directional. This operation is denoted with the hat operator ^.
For 2D points and directions, we have:
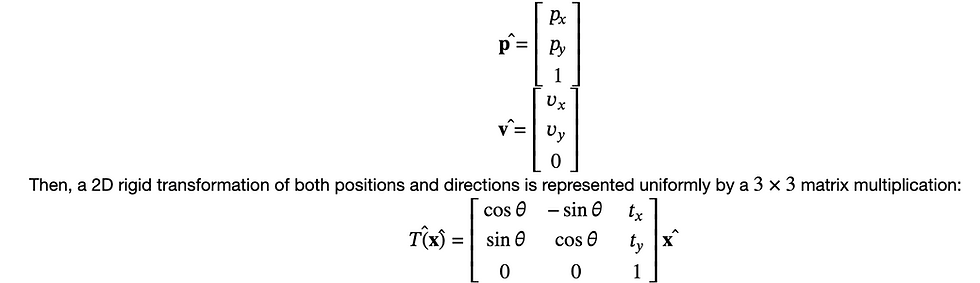
where the original transformation đ(đą)=đ (đ)đą+đ is a rotation about angle đ followed by a translation of vector đ=(đĄđĽ,đĄđŚ).
In 3D, the hat operator adds a 4th coordinate: For 2D points and directions, we have
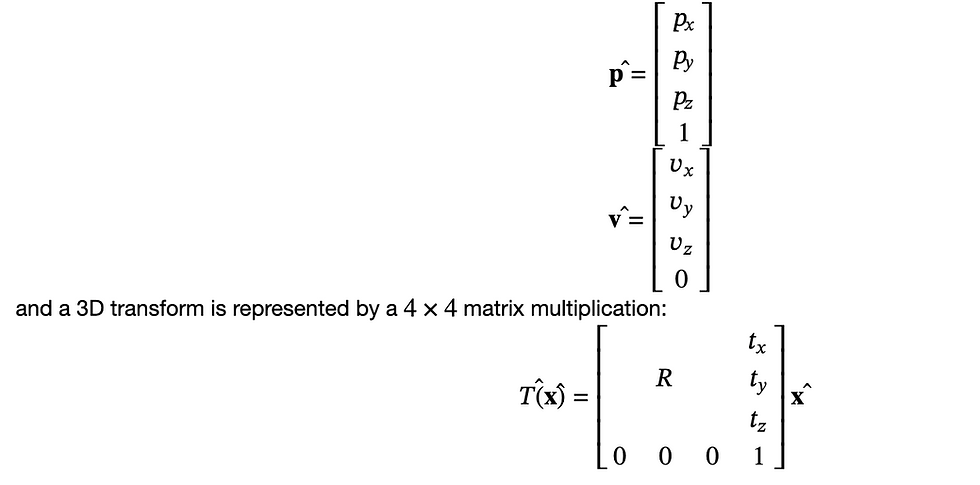
Note that when applied to homogeneous positions, the rigid transform is applied to the first two coordinates of the vector while the homogeneous coordinate remains 1 (since the dot product of a position representation with the last row of the matrix is 1). Also, when applied to homogeneous directions, only the rotation is applied to the first two coordinates of the vector, since the third 0 coordinate nullifies the effect of the third column. The homogeneous coordinate remains 0, since the dot product of a position representation with the last row of the matrix is 0.
The nice thing about this representation is that transform application is a matrix-vector multiply, transform composition is a matrix-matrix multiply, and transform inversion is a matrix inversion. This makes it much easier to write out complex transformations. For example, consider the problem of the coordinate transform from frame đ´ to frame đľ that we described above. Rather than writing out the operator expression
đâ1đľ(đđ´(đŠđ´)), using homogeneous coordinates this becomes a series of matrix-matrix and matrix-vector multiplies:
đĚ đľ=đĚ â1đľâ đĚ đ´â đĚ đ´.
3. Summary
Key takeaways:
Coordinates are numerical representations of geometric concepts, like points, directions, frames of reference, and movement.
Points, directions, and displacements in đ-dimensional space are represented by đ-dimensional vectors, while rotations and scalings are represented by đĂđ matrices.
Rigid transformations consist of a rotation followed by a translation. They represent both rigid body movement and changes of coordinate frame.
Homogeneous coordinates represent rigid transforms using matrix multiplication in an đ+1 dimensional space where the last coordinate is either 0 or 1.
When working with coordinates it is easy to make mistakes. Having clear assumptions, clear notation and/or using coordinate management software can reduce the risk of error.
Until next time, keep your eyes on the horizon as we venture into new frontiers in the mesmerizing realm of robotics.
#RoboByte #RoboticsWorld #Innovation #roboticswithpruthvi #AI #HumanRobotInteraction #ComputerVision #MachineLearning #RealWorldApplications #RoboticsImpact #BlogPost #RoboticsExploration #RoboticsKinematics #CoordinateFrames #RobotMotion #RoboticsSeries #LearningTogether #RoboticsJourney #LinkedInCommunity #Innovation #TechnologyRevolution #Introtorobotics #Robotics #Innovation #technology
Comments